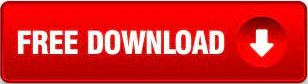
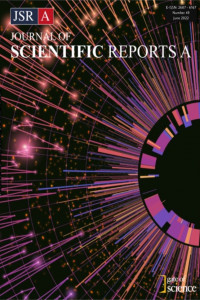
Deep learning refers to techniques that build on developments in artificial neural networks in which multiple network layers are added to increase the levels of abstraction and performance 8. In the field of general imaging and computer vision, deep learning is the leading machine learning tool 7.

It enables computers to find hidden insights without being explicitly programmed using algorithms that iteratively learn from the data 5, 6. Machine learning is an application of artificial intelligence (AI). Failure to identify implants preoperatively resulted in additional requested implants, added surgical time, increased perioperative morbidity, and increased healthcare cost 3, 4. 10% of implants could not be identified preoperatively with 2% not being identified intraoperatively. A recent survey of arthroplasty surgeons showed that surgeons spent approximately 20 min for each revision case to identify the implant preoperatively. One of the critical steps in preoperative planning for revision of total hip arthroplasty is the identification of the failed implant. Projections based on population studies pointed to continued increases in the prevalence of revision procedures 2. The increasing absolute number of primary arthroplasties, expansion of the indications to include younger and more active individuals, are all likely to contribute to overall revision rates. The etiology of the increase in the number of revision procedures is multifactorial. However, despite the clinical success of THAs, the number of revision THAs performed has increased with time. Total hip arthroplasty (THA) has been called the “Operation of the Century” because of its durability, reliability, and reproducibility in relieving pain and improving function in patients with coxarthrosis 1. Using stem images and cup images together is not more effective than using images from only one perspective. Using stem images or cup images to train the CNN can both achieve effective identification accuracy, with the accuracy of the stem images being higher. This technology could offer a useful adjunct to the surgeon in preoperative identification of the prior implant.
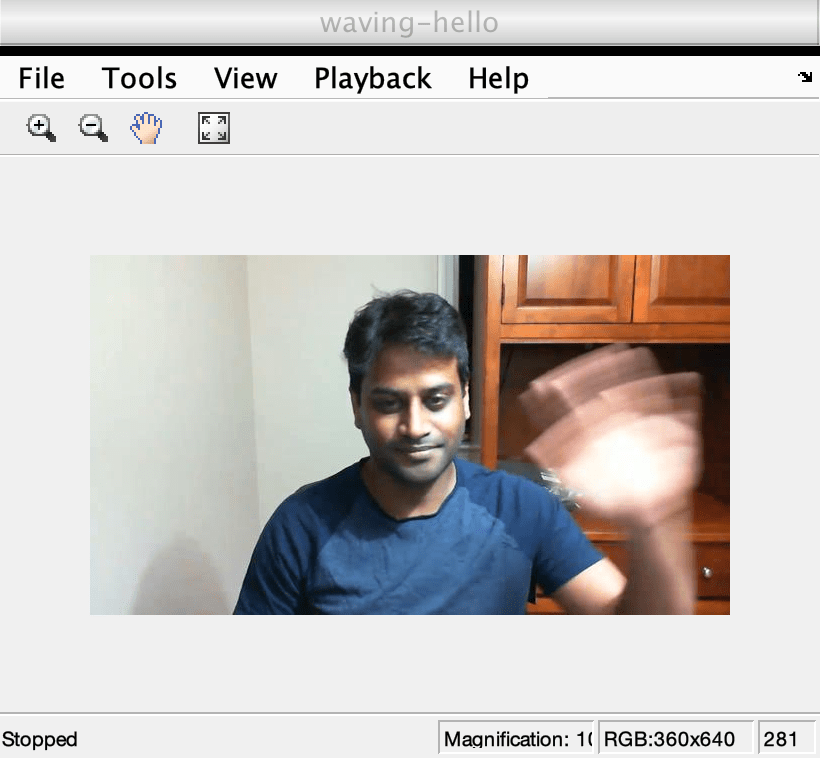
CNN can accurately distinguish between specific hip arthroplasty designs. Our results showed that jointly using stem images and cup images did not improve the classification accuracy of the CNN model. After 1000 training epochs the model classified 4 implant models with very high accuracy. The training and validation data sets were comprised of 357 stem images and 357 cup radiographs across 313 patients and included 4 hip arthroplasty implants from 4 leading implant manufacturers. We also evaluate the difference in performance of models trained with the images of the stem, the cup or both. Performance was compared with the operative notes and crosschecked with implant sheets. We harness the pre-trained ResNet50 CNN model and employ transfer learning methods to adapt the model for the implants identification task using a total of 714 radiographs of 4 different hip arthroplasty implant designs. In this study, we propose a novel deep learning-based approach to identify hip arthroplasty implants’ design using anterior–posterior images of both the stem and the cup. The purpose of this study was to develop and evaluate the performance of deep learning methods based on convolutional neural networks (CNN) to detect and identify specific hip arthroplasty models.
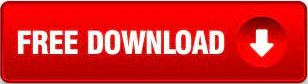